Solar Power Forecasting
As the world increasingly turns to renewable energy sources, solar power has become a key player in our global energy strategy. But unlike traditional power generation methods, solar energy is inherently variable—it depends on sunlight, which fluctuates throughout the day and is affected by weather conditions. This variability can pose significant challenges for maintaining a stable power grid. That’s where solar power forecasting comes in. This blog will explore what solar power forecasting is, why it’s essential, how it’s done, the challenges involved, and the steps needed to improve this critical process.
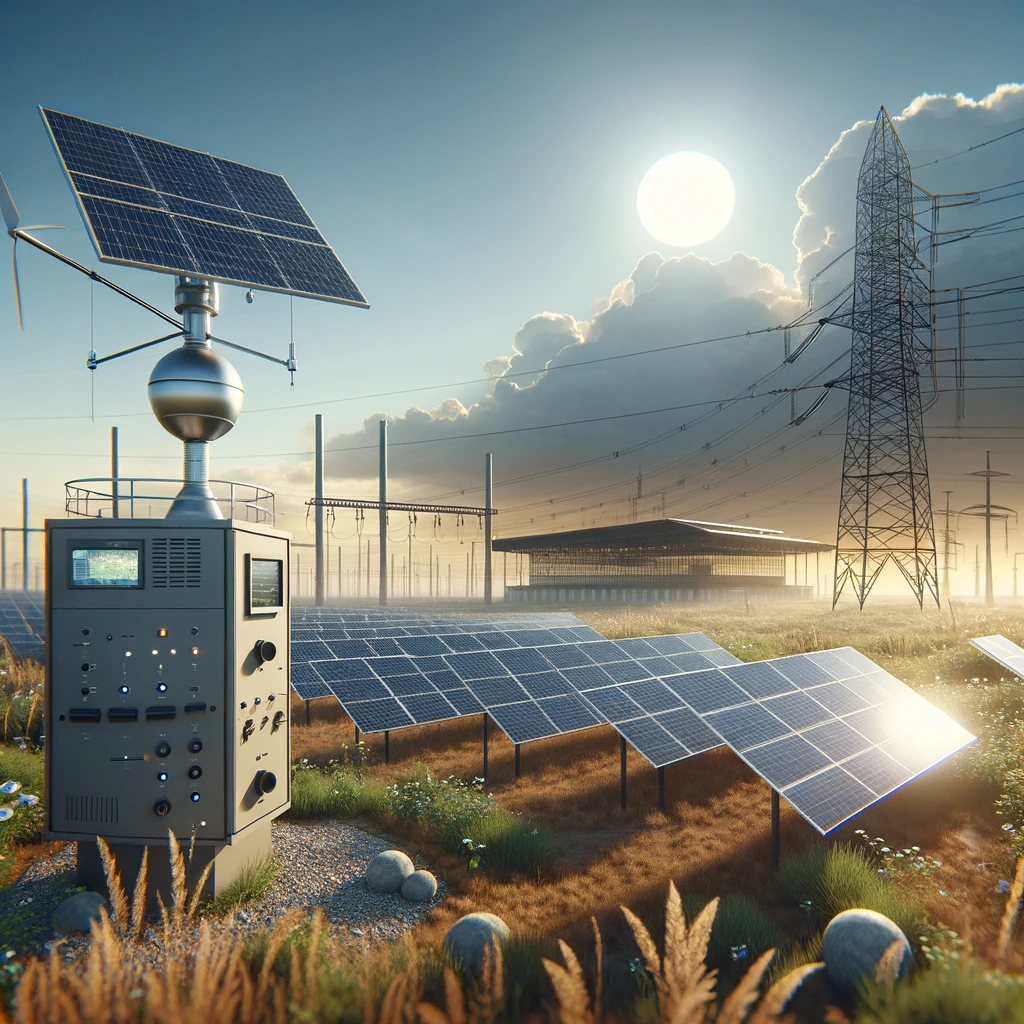
Why is Solar Power Forecasting Important?
Imagine you’re running a power grid. Your job is to ensure that the amount of electricity generated matches the demand at all times. If too much power is generated, it can overload the system; if too little, it can cause blackouts. With traditional energy sources like coal or natural gas, you can control the output as needed. But with solar power, you’re at the mercy of the weather—cloud cover, rain, and the time of day all affect how much electricity solar panels can produce.
Here’s where solar power forecasting becomes crucial. By accurately predicting how much solar power will be generated at different times, grid operators can plan ahead. For instance, if a cloudy day is forecasted, they might ramp up other power sources to make up for the shortfall in solar energy. Conversely, if a sunny day is expected, they can rely more on solar power and reduce reliance on fossil fuels. This balancing act is essential for maintaining grid stability and ensuring that we can safely and efficiently integrate more renewable energy into our power systems.
How Solar Power Forecasting Works
Solar power forecasting isn’t just about looking at the weather forecast and making a guess. It involves a detailed process with several stages, each crucial to ensuring accurate predictions.
Data Collection and Analysis:
- Weather Data: This includes information on sunlight intensity (solar irradiance), cloud cover, temperature, and wind speed. For example, if a region is expected to experience heavy cloud cover, the forecast will account for reduced sunlight and therefore predict lower solar power generation.
- Plant Configuration: Details about the solar plant itself, such as the type and orientation of the solar panels, are considered. A plant with panels that track the sun’s movement might generate more power than one with fixed panels, especially in the morning and evening.
- Historical Performance: Past data on how much power the plant has generated under similar conditions is also factored in. For instance, if a certain weather pattern previously resulted in a 20% reduction in power output, that information helps fine-tune the forecast.
Solar Power Scheduling:
- Once the forecast is made, a schedule is created that outlines how much solar power is expected to be available at different times of the day. This schedule is crucial for grid operators, who use it to plan how they will balance the power supply with demand. For example, if the schedule shows a peak in solar power around noon, the grid operator might decide to reduce output from gas-fired plants at that time.
- Once the forecast is made, a schedule is created that outlines how much solar power is expected to be available at different times of the day. This schedule is crucial for grid operators, who use it to plan how they will balance the power supply with demand. For example, if the schedule shows a peak in solar power around noon, the grid operator might decide to reduce output from gas-fired plants at that time.
Submission to Grid Operators:
- The final schedule is submitted to the Regional Load Dispatch Center (RLDC), which manages the grid’s operations. This allows the grid operators to make necessary adjustments in real-time. If the forecast indicates a sudden drop in solar power due to an unexpected weather change, the RLDC can quickly bring other power sources online to compensate.
Regulatory Framework Governing Solar Power Forecasting
In countries like India, where solar power is rapidly expanding, the process of forecasting and scheduling is regulated to ensure consistency and reliability. The Central Electricity Regulatory Commission (CERC) oversees these regulations. For instance, any deviation between the forecasted and actual power generation needs to be managed through a commercial mechanism. If a solar plant generates less power than predicted, it may face penalties under these regulations.
This regulatory framework helps ensure that all players in the energy market—from solar power generators to grid operators—are aligned in their efforts to maintain a stable power supply. It also incentivizes solar power generators to improve the accuracy of their forecasts, as errors can lead to financial penalties.
Challenges in Solar Power Forecasting
Despite the advancements in forecasting techniques, several challenges make accurate solar power forecasting difficult.
Abrupt Weather Changes:
- Weather can change suddenly and unexpectedly. For example, localized cloud formations that are not captured by broader weather models can lead to significant drops in solar power output. If a large cloud suddenly covers a solar farm, the amount of generated power could drop by 50% or more within minutes, making it challenging for the grid to adapt quickly.
- Weather can change suddenly and unexpectedly. For example, localized cloud formations that are not captured by broader weather models can lead to significant drops in solar power output. If a large cloud suddenly covers a solar farm, the amount of generated power could drop by 50% or more within minutes, making it challenging for the grid to adapt quickly.
Infrastructure Limitations:
- Accurate forecasting requires a robust network of weather stations and radars to gather real-time data. However, in many regions, these infrastructures are insufficient. For example, in India, the locations of Automated Weather Stations (AWS) are not always aligned with renewable energy zones, which limits the accuracy of the data they collect. Additionally, there aren’t enough radars to cover all the areas where solar plants are located, leading to gaps in the data.
- Accurate forecasting requires a robust network of weather stations and radars to gather real-time data. However, in many regions, these infrastructures are insufficient. For example, in India, the locations of Automated Weather Stations (AWS) are not always aligned with renewable energy zones, which limits the accuracy of the data they collect. Additionally, there aren’t enough radars to cover all the areas where solar plants are located, leading to gaps in the data.
Variability in Solar Irradiance:
- Solar irradiance—the amount of sunlight hitting the earth’s surface—can vary significantly even between two nearby locations. For example, two solar plants in the same region might experience different levels of sunlight due to microclimates or localized weather conditions. This variability makes it difficult to predict power output accurately across a wide area.
Examples of Forecasting Errors and Their Impact
To illustrate these challenges, consider a large solar farm that was expected to generate 250 MW of power on a given day. However, due to a sudden formation of low-level clouds, the actual generation dropped to 200 MW. If the grid was relying on that 50 MW of power, the shortfall could lead to voltage fluctuations or even blackouts if backup sources are not activated quickly enough. Moreover, under the CERC’s regulations, the solar farm might incur a financial penalty for not meeting its forecasted output.
Way Forward: Improving Solar Power Forecasting
To address these challenges, several strategies can be employed:
Enhanced Weather Forecasting Models:
- Current weather models need to evolve from focusing on larger regional forecasts (meso-scale) to more localized, detailed forecasts (micro-scale). This means investing in new technologies and methodologies that can capture small-scale weather events, such as localized cloud cover or fast-moving weather fronts.
- Current weather models need to evolve from focusing on larger regional forecasts (meso-scale) to more localized, detailed forecasts (micro-scale). This means investing in new technologies and methodologies that can capture small-scale weather events, such as localized cloud cover or fast-moving weather fronts.
Infrastructure Development:
- Increasing the number of AWS and radars, especially in areas with high concentrations of solar farms, is crucial. For example, adding more weather stations in regions like Ananthapuram Solar Park in India would provide more accurate and site-specific data, improving forecast accuracy.
- Increasing the number of AWS and radars, especially in areas with high concentrations of solar farms, is crucial. For example, adding more weather stations in regions like Ananthapuram Solar Park in India would provide more accurate and site-specific data, improving forecast accuracy.
Leveraging Advanced Technologies:
- Incorporating artificial intelligence (AI) and machine learning (ML) into forecasting models can significantly improve their accuracy. AI can analyze vast amounts of data from various sources in real time, detecting patterns and predicting abrupt changes in solar irradiance that traditional models might miss. For instance, AI could be used to analyze satellite images and predict cloud movements that could affect solar generation.
- Incorporating artificial intelligence (AI) and machine learning (ML) into forecasting models can significantly improve their accuracy. AI can analyze vast amounts of data from various sources in real time, detecting patterns and predicting abrupt changes in solar irradiance that traditional models might miss. For instance, AI could be used to analyze satellite images and predict cloud movements that could affect solar generation.
Collaboration Among Stakeholders:
- Improving solar power forecasting is a collective effort. Government agencies, renewable energy developers, and weather forecasting agencies need to work together to share data and improve models. For example, the Indian Meteorological Department (IMD) could collaborate with solar power companies to provide more accurate weather forecasts tailored to the needs of the renewable energy sector.
Conclusion
Solar power forecasting is a vital tool for integrating renewable energy into our power grids. While the process is complex and faces significant challenges, advancements in technology and infrastructure, coupled with effective collaboration among stakeholders, can lead to more accurate forecasts. As we continue to rely more on renewable energy sources like solar, improving our ability to predict and manage solar power generation will be essential for ensuring a stable and sustainable energy future.